You’ve been hearing the term “AI governance” more and more. It’s showing up in board meetings, vendor pitches, LinkedIn posts. But every time you ask what it actually means, the answers get vague. Something about ethics, or models, or data. And you’re left wondering—is this just the same thing as data governance with a shinier label? Or is there something genuinely new I need to worry about?
You’re not alone. For most execs and team leads, this conversation feels like a moving target. You’re already wrestling with data quality issues, compliance risks, and the sheer scale of your information landscape. Now AI is being layered on top—and no one’s giving you a straight answer about how governance actually works in this new world.
That’s what this article is here to do.
At Alchemy, we’ve worked with organisations across sectors to untangle the chaos of data governance and build something practical, strategic, and scalable. We’ve seen firsthand how the rise of AI has added confusion—but also clarity, once you understand where the lines are.
In this article, you’ll get a clear, no-jargon breakdown of what data governance and AI governance really mean, how they overlap, how they differ—and what you need to do next to stay ahead.
What is Data Governance?
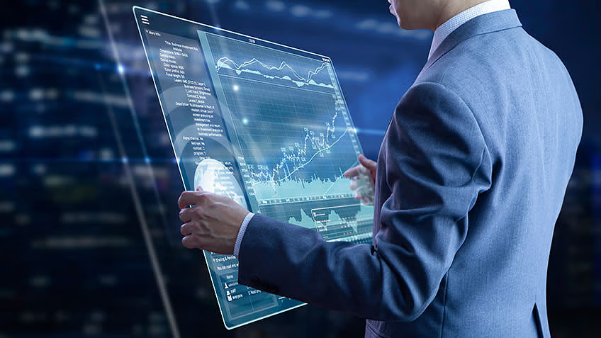
Did you know that Data Governance is a top priority for 65% of leaders?
Think of data governance as the rulebook for how your organisation handles data. It’s the policies, roles, processes, and standards that ensure your data is accurate, secure, and used responsibly.
At its core, data governance answers questions like:
- Who owns which data?
- What rules apply to how data is stored and shared?
- How do we ensure the data we use is reliable?
- What do we do if something goes wrong?
For many organisations, data governance starts with cleaning up chaos—spreadsheets scattered across teams, systems that don’t talk to each other, or reports based on outdated info. But mature data governance goes further. It helps you unlock trusted insights, comply with regulations like the Australian Privacy Principles or APRA standards, and reduce risk exposure from data misuse.
The key pillars typically include:
- Data quality – Making sure your data is complete, consistent, and trustworthy.
- Data ownership – Assigning clear roles and responsibilities.
- Policies and standards – Setting rules for how data is used and managed.
- Compliance and security – Ensuring data is protected and meets legal requirements.
It’s not just a tech thing. Strong data governance supports smarter decisions, smoother operations, and—crucially—the ability to scale things like automation and AI with confidence.
What is AI Governance?
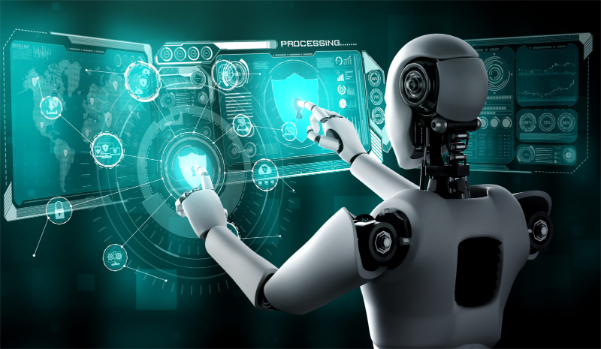
If data governance is about managing your data, AI governance is about managing how artificial intelligence is used in your organisation.
It’s the framework that makes sure AI systems are developed, deployed, and monitored in a way that is ethical, transparent, and aligned with your business goals and values. It goes beyond just the technical performance of AI—it asks whether your AI is fair, explainable, accountable, and lawful.
AI governance typically covers:
- Ethical principles – Are the algorithms making decisions that are fair and unbiased?
- Transparency – Can someone explain how an AI decision was made?
- Accountability – Who is responsible if the AI gets it wrong?
- Risk management – Are you monitoring for unexpected outcomes or misuse?
- Regulatory compliance – Are your AI practices in line with current or upcoming laws?
Unlike data governance, which is often internal-facing and operational, AI governance is much more public-facing and risk-sensitive. It touches not just IT and compliance, but also legal, marketing, HR, and executive teams—anyone who might be impacted by or responsible for AI decisions.
And here’s the kicker: you can’t have AI governance without good data governance. If your data is biased, incomplete, or poorly governed, any AI you build on top of it will inherit those issues—and potentially amplify them.
Why the Confusion Between Data Governance & AI Governance?
On paper, the difference between data governance and AI governance seems straightforward. But in practice? The lines blur fast—and here’s why.
First, AI runs on data. So it’s easy to assume that if you’re “governing the data,” then you’re automatically governing the AI too. But that’s only part of the story. AI brings new layers of complexity—like algorithmic bias, model drift, explainability—that sit on top of your data foundations. They need their own rules, oversight, and accountability.
Second, a lot of vendors and consultants use the terms interchangeably—often because they’re selling one while talking about the other. So you’ll hear people talk about “AI governance” when they’re actually referring to data catalogues, lineage tools, or privacy compliance. Or they’ll package data governance features under an “AI governance” label because it sounds more futuristic.
And finally, organisations often struggle to separate them because the responsibilities overlap. Who’s in charge of data quality? Who reviews AI risks? Who talks to regulators? In many cases, it’s the same few people juggling both—and without clear definitions, the governance conversation turns into a game of buzzword bingo.
That’s why it’s so important to draw the distinction. You can’t govern AI effectively without governing your data—but managing your data isn’t enough to manage your AI. They’re connected, but they’re not the same.
Do You Need Both Data Governance and AI Governance?
Short answer: yes—but not necessarily at the same time, or with the same intensity.
If your organisation is still struggling to get basic data governance in place—clarifying data ownership, improving data quality, managing compliance—then that’s your first priority. Without trusted, well-managed data, any AI initiative you launch is going to be shaky at best, risky at worst.
But if you’re already using AI—or planning to—you’ll need to layer AI governance on top. Not just to manage risk, but to ensure your AI systems actually deliver value without unintended consequences. That means thinking beyond just data and asking questions like:
- Is the AI output explainable to stakeholders?
- Are we testing for bias and fairness?
- Do we have a human in the loop for critical decisions?
- Are we logging decisions in case we’re audited?
So no, this isn’t an either/or. It’s more of a maturity journey:
- Start with solid data governance – so you can trust your inputs.
- Build toward AI governance – as you scale your use of AI and automation.
Trying to jump into AI governance without stable data governance is like installing a satellite dish on a crumbling roof. You might get a signal, but you won’t want to stand under it.
How Solid Data Governance Supports AI Governance
Think of data governance as the foundation, and AI governance as the structure you build on top. If the foundation is shaky, the whole thing is at risk.
Here’s how strong data governance directly supports your AI governance efforts:
- Bias mitigation starts with data quality: If your datasets are skewed or inconsistent, your AI models will be too. Good data governance helps you identify and correct these issues early.
- Traceability and auditability: When your data is properly catalogued and documented, it’s much easier to explain how your AI made a decision—because you can trace it back to the inputs.
- Compliance readiness: Regulations like the GDPR, the Australian Privacy Principles, and emerging AI-specific laws expect organisations to show how data is used in automated decisions. Data governance gives you the tools to do that.
- Clear accountability: Data governance assigns ownership—who’s responsible for what data. That clarity extends to AI, where you’ll need defined roles for things like model oversight and risk reviews.
- Model monitoring and maintenance: AI systems degrade over time as data changes. With good governance, you’ve already got processes in place to monitor data drift, which helps you manage model drift too.
In short, AI governance doesn’t replace data governance—it relies on it. If your data house isn’t in order, you’ll spend most of your AI energy cleaning up messes instead of driving value.
What to Do Next
If you’re unsure where to start, focus on the one thing that will support everything else: your data.
Here’s a practical path forward:
- Assess your current state
Do you know who owns your data? Can you trust it? Are there clear policies in place for how it’s handled and shared? If not, that’s your first gap to close.
- Align governance with business goals
Whether it’s compliance, operational efficiency, or AI innovation—your governance approach should be driven by what the business is trying to achieve. Not the other way around.
- Start building your governance framework
Use established best practices like the DAMA-DMBOK to define roles, policies, and standards. Don’t try to solve everything at once. Start small and scale.
- Identify where AI is in play—or coming soon
Are you already using AI tools? Planning to? Map out where AI systems are (or will be) used, and who’s responsible for them. This gives you a starting point for adding AI governance without overwhelming your teams.
- Educate and engage stakeholders
Governance isn’t just for IT. Product managers, legal teams, compliance officers, and execs all play a part—so bring them into the conversation early.
Remember: you don’t need to “solve governance” overnight. You just need to take the next smart step. And often, that starts with asking: do we know what’s going on with our data?