Let’s get one thing straight—AI doesn’t fail because of the technology. It fails because data is a mess, and that usually means there’s no solid AI data strategy behind it.
Across industries, organisations are pouring money into AI pilots, tools, and talent. But when the results don’t match the hype, it’s usually not because the models are broken. It’s because they’re built on shaky foundations. According to KPMG’s recent report Trust, attitudes and use of Artificial Intelligence: A global study 2025, concerns around data quality and trust remain among the most significant barriers to AI adoption.
If your data is fragmented, outdated, or poorly governed, no amount of AI investment will deliver real value. And if you’re not aligning your data efforts with business goals, you’re just building expensive toys.
At Alchemy Solutions, we’ve seen the same pattern again and again: successful AI doesn’t start with models—it starts with methodical, disciplined data practices. In this article, you’ll learn the seven best practices for building a rock-solid AI data strategy that actually moves the needle—from data architecture to quality, governance, and measurement.
Why Every AI Data Strategy Starts with Strong Data Foundations
If your data is shaky, your AI won’t stand a chance.
It sounds obvious, but it’s one of the most overlooked truths in AI adoption. Businesses get excited about advanced models, automation, and generative AI—but skip the boring (yet essential) groundwork. The result? Expensive failures, stalled projects, and leadership frustration.
Here’s why strong data foundations are the bedrock of any effective AI data strategy:
1. AI Is Only as Good as the Data It Learns From
Bias, gaps, or inconsistencies in your data directly affect AI outcomes. If your source data is flawed, the insights and outputs will be too—no matter how advanced your model is.
2. Data Must Be Timely, Trusted, and Accessible
Real-time decisions need real-time data. If your AI tools can’t access accurate data fast, you’re not driving value—you’re just analysing history.
3. Architecture Drives Agility
Your AI data strategy has to account for how data flows between systems. Without the right architecture—one that integrates structured and unstructured sources—AI initiatives get bogged down by silos and manual workarounds.
4. Compliance Can’t Be an Afterthought
In a world of growing data regulation, embedding compliance into your AI data strategy from day one is essential. If you don’t, you’re opening the door to security risks, audits, and reputation damage.
In short: before you talk AI, fix your data. The strongest strategies are built on quality, integration, governance, and speed.
7 Best Practices for a Future-Proof AI Data Strategy
Building a strong AI data strategy isn’t about doing everything at once. It’s about focusing on the key areas that make your data usable, trustworthy, and strategically aligned.
Here are seven proven best practices to help you build a foundation that AI can actually thrive on:
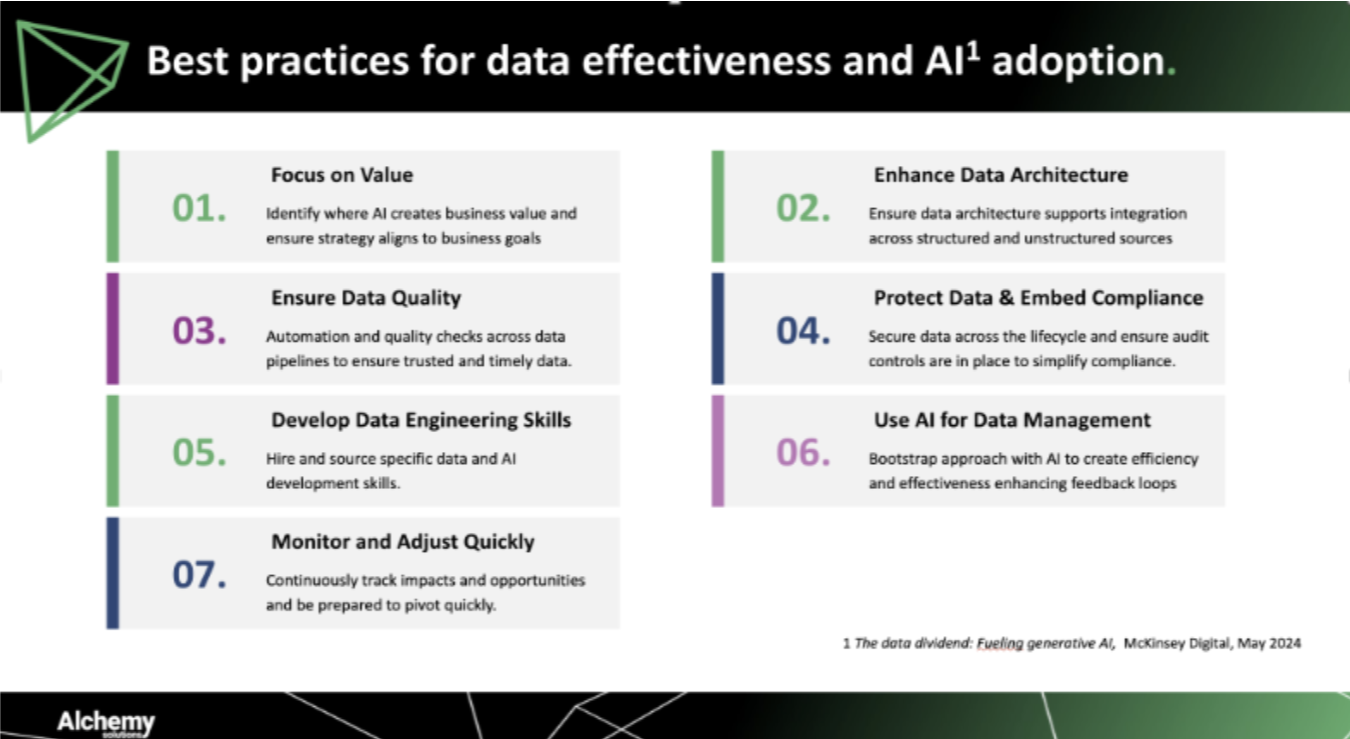
1. Focus on Value First
Start by identifying where AI can drive the most business impact. Too many organisations dive into AI without linking it to clear goals. Your strategy should always begin with this question: How will this support real business outcomes?
2. Enhance Your Data Architecture
Ensure your systems support seamless integration across structured and unstructured data. A scalable, flexible architecture is what turns scattered data into usable insight.
3. Ensure Data Quality at Every Stage
AI models need clean, accurate, and consistent data. Automate quality checks across your data pipelines to ensure reliability and reduce manual errors.
4. Protect Data and Embed Compliance
Security and compliance must be built into your AI data strategy from the ground up. This means protecting data across its lifecycle and embedding audit controls to meet regulatory standards.
5. Develop Data Engineering Skills
You need the right talent to execute your strategy. Invest in people who understand both the technical side of data and the needs of your business. Without the right skills, even the best tools fall flat.
6. Use AI for Smarter Data Management
Here’s where the cycle improves itself: use AI to streamline data processes, create feedback loops, and drive more efficiency into your workflows. Think of it as using AI to power the strategy behind your AI.
7. Monitor and Adjust—Fast
Your AI data strategy isn’t static. Track performance, gather feedback, and pivot quickly when the data tells you something isn’t working. Agility is just as important as accuracy.
These best practices are your blueprint for transforming AI from a buzzword into a business advantage.
How to Fix a Broken or Outdated AI Data Strategy
Let’s be honest—most organisations didn’t get their AI data strategy right the first time. If your efforts feel stuck, disjointed, or like they’ve lost momentum, you’re not alone. The good news? It’s fixable.
Here’s how to course-correct and build a smarter, more resilient AI data strategy:
1. Audit What You’ve Already Got
Before rebuilding, assess your current data estate. Where are the gaps? What’s duplicated, siloed, or outdated? A clear view of your baseline makes it easier to prioritise what needs fixing.
2. Reframe Your Strategy Around Business Outcomes
Move away from tech-first thinking. Align your AI data strategy with tangible business goals—whether it’s reducing costs, speeding up processes, or improving customer experience. This ensures stakeholder buy-in and keeps your efforts on track.
3. Break the Work into Manageable Phases
You don’t need to transform everything overnight. Focus on one domain or use case where you can deliver value quickly. Prove it works, then scale. This iterative approach builds confidence and momentum.
4. Strengthen Governance and Ownership
One common failure point? No one truly owns the data. Assign clear roles and responsibilities for governance, quality, and usage. This keeps your AI data strategy accountable and sustainable.
5. Choose Partners That Drive Results, Not Hype
If internal capability is thin, bring in external expertise—but look for partners who prioritise outcomes, not just implementation. They should help you build internal capability, not create dependency.
Fixing your AI data strategy isn’t about starting from scratch—it’s about being intentional, focused, and brutally honest about what’s not working.
Building a Smarter AI Data Strategy That Delivers ROI
AI isn’t a silver bullet. But with the right data strategy, it can be a serious competitive advantage.
Too many businesses race toward AI adoption without first getting their data house in order. The result? Disconnected pilots, wasted spend, and zero impact. But when your AI data strategy is rooted in value, governance, and scalable architecture, everything changes.
You move from experimentation to execution.
From hype to measurable outcomes.
From fragmented data to unified insight.
The path to real ROI starts with clarity—and a plan. Whether you’re refining your current approach or starting from scratch, the best time to fix your data foundations is before your AI ambitions outpace your infrastructure.
Want help turning your AI data strategy into business results?
Let’s talk. Alchemy Solutions can help you identify the gaps, design a scalable roadmap, and build the data muscle your organisation needs to lead with AI. You can also explore our in-depth guide on Challenges in Building AI Readiness and How Alchemy Solutions Can Help. It offers practical insights that build on the ideas covered in this article.